Estimation of overdamped model¶
First download the data and load the trajectories. Don’t forget to adapt the location of the file
[ ]:
import numpy as np
import folie as fl
data = fl.Trajectories(dt=1.0e-3)
n=1 # Let's use the first molecule.
trj = np.loadtxt(f"DATA")
data.append(trj.reshape(-1,1))
print(data) #Let's check what we have
Then define a model, here we are going to use the default 1D overdamped model. We can then fit the model. To start we use a simple KramersMoyal estimation
[2]:
domain = fl.MeshedDomain.create_from_range(np.linspace(data.stats.min , data.stats.max , 10).ravel())
model = fl.models.OverdampedSplines1D(domain=domain)
estimator = fl.KramersMoyalEstimator(model)
model = estimator.fit_fetch(data)
We can then plot the force and diffusion profile
[6]:
import matplotlib.pyplot as plt
xfa = np.linspace(np.min(trj),np.max(trj),75)
fig, ax = plt.subplots(1, 1)
# Force plot
ax.set_title("Force")
ax.set_xlabel("$x$")
ax.set_ylabel("$F(x)$")
ax.grid()
ax.plot(xfa, model.drift(xfa.reshape(-1, 1)))
[6]:
[<matplotlib.lines.Line2D at 0x7fdeb7139540>]
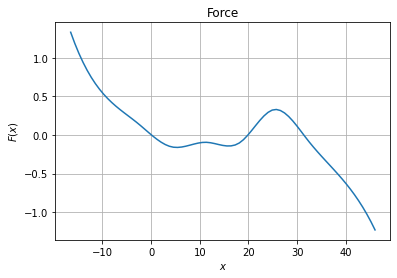
[4]:
fig, ax = plt.subplots(1, 1)
# Diffusion plot
ax.set_title("Diffusion")
ax.grid()
ax.set_xlabel("$x$")
ax.set_ylabel("$D(x)$")
ax.plot(xfa, model.diffusion(xfa.reshape(-1, 1)))
[4]:
[<matplotlib.lines.Line2D at 0x7fdeb7223f70>]
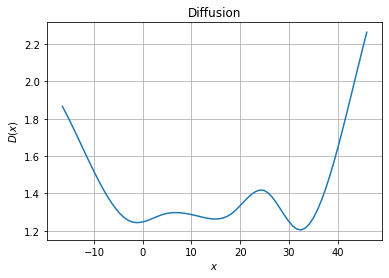
But also obtain the free energy profile. The free energy profile \(V(x)\) is related to the force \(F(x)\) and the diffusion \(D(x)\) from
The relation can then be inverted to obtain the free energy profile.
[5]:
pmf=fl.analysis.free_energy_profile_1d(model, xfa)
fig, ax = plt.subplots(1, 1)
# Diffusion plot
ax.set_title("PMF")
ax.grid()
ax.set_xlabel("$x$")
ax.set_ylabel("$\\beta U(x)$")
ax.plot(xfa, pmf)
[5]:
[<matplotlib.lines.Line2D at 0x7fdeb72c21a0>]
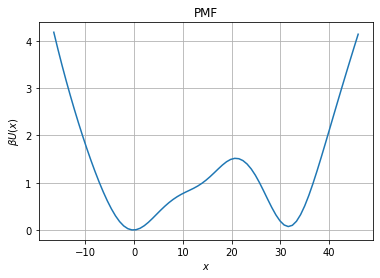
Since there is two well, we can also compute the mean first passage time to go from point x to 0.
[1]:
x_mfpt, mfpt = fl.analysis.mfpt_1d(model_simu, 0, [-20.0, 50.0], Npoints=500)
fig, ax = plt.subplots(1, 1)
# MFPT plot
ax.set_title("MFPT from x to 0")
ax.grid()
ax.set_xlabel("$x$")
ax.set_ylabel("$MFPT(x,0)$")
ax.plot(x_mfpt, mfpt)
---------------------------------------------------------------------------
NameError Traceback (most recent call last)
Cell In[1], line 1
----> 1 x_mfpt, mfpt = fl.analysis.mfpt_1d(model_simu, 0, [-20.0, 50.0], Npoints=500)
NameError: name 'fl' is not defined
Parallel execution¶
See https://scikit-learn.org/stable/computing/parallelism.html for an overview of available options.
Likelihood estimator are parallelized using n_jobs= with computation of likelihood being parallelized over trajectories.